What I Learned at Intel Innovation 2022
Silicon Valley is a world famous hotspot for innovation, where all the biggest tech companies are headquartered. I was happy to be back there again as a geek and participant in one of the biggest tech events this year – Intel Innovation 2022.
As an event made for developers by developers, it was all about the latest ideas and breakthroughs, where innovators share open ecosystem resources and visitors can experience brand new solutions in person. The most exciting part for me was the chance to meet many of my colleagues for the first time, after more than 2 years of COVID lockdown.
As an Intel partner, we showcased our AI Playground concept in Booth 343, which delighted many visitors in the way it made AI friendly, educational, and fun.
Meeting tech heroes Pat Gelsinger and Linus Torvalds
Intel CEO Pat Gelsinger delivered the keynote on Day 1 that included a tribute to Linus Torvalds, creator of Linux, which is considered as the backbone for many platforms in use today. It is very inspiring for me to see two computer science giants on one stage, and witness the respect they clearly have for each other’s work.
Linus was telling Pat that he lost his copy of 8386, which started him on his journey as a developer. Pat then gave a signed copy to him, and proceeded to honor him with the first Intel Innovation Award for Lifetime Achievement. Watching that moment, I felt like I was at university again taking apart motherboards. I never imagined I’d get to be in the same room with the people that created it.
I have been a longtime fan of Linus as an open source champion, which allows people like me to develop and build solutions on top of what others have done. His advocacy for open discussions resonates with me deeply, where engineers do not only execute systems, but are part of the decision making process and become invested in its outcomes. I really believe that innovation can only thrive where the developer community is a true community of people working together and getting things done.
Pat’s promise to that community is this:
“Developers – whether software or hardware focused – you see the future. Our job at Intel is to open that world up to you. Together we've peeked into the future, one we will create together.”
Later, Pat dropped by our booth and spent a bit of time playing a Lego version of himself driving to Mars.
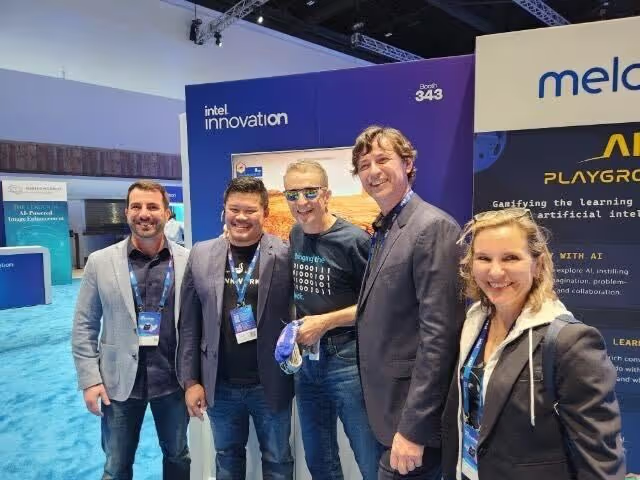
It was a very proud moment for us to geek out with Pat, who can discuss ideas at a very low level, highly technical topics. We got to speak to him in more detail about the great work Intel is doing to empower ISVs like us, with technologies like OpenVINO and OVMS, NUC, and Geti. These are some of the key ingredients that allow us to create products like Viana™ and solutions like AI Playground.
Conversation with Andrew Ng on democratizing AI
Just when I thought things couldn’t get any better, I met Andrew Ng on Day 2 after his very inspiring presentation. He is the CEO and founder of Landing AI, and a living legend in the AI community.

I was running a bit late that day, but I was glad I didn’t miss Andrew’s keynote on democratizing AI.
“I hope someday, even the owner of a small pizzeria, who does have data, can use AI to drive the performance of their own small business.” Andrew Ng
I agree and believe that AI cannot reach its full potential until it’s accessible to everyone. AI needs to involve more people and be made available to those outside the tech industry. But in order for it to innovate more, it must reach more people, and more industries.
In my conversation with him afterwards, I had the chance to share our own development process at meldCX. I also learned a lot about how his own team at Landing AI runs their machine learning development, with data as front and center. Some of my key takeaways are:
1. Cycle in minutes not months.
Machine learning is a cycle of execution – from dataset preparation to annotation to model training to inspection of results – in which you continually improve the dataset and repeat the process again.
According to Andrew, it's more about debugging than development. You can get very good results if you can work through the ML process quickly, rather than spending months producing your first proof of concept. Spend just a few days, or even minutes, to build the first version of your ML model, then iterate quickly by getting into the “debugging loop” much faster. With that mindset, you may expect to build a model that’s not going to work the first time, but you can then find systematic ways to debug it. In Andrew’s experience, teams that use this approach end up making much faster progress.
This validates our current practice of model training and iteration. In our team, we call this “project momentum” where we become more effective by isolating problematic training results one at a time. Then we quickly test, and move to the next cycle, ensuring that each time we are deploying a better model than the one before.
2. Focus on the data, not just the model.
Andrew’s advocacy is data-centric AI, which promotes data as the most important component in machine learning. You can significantly improve accuracy and performance just by improving the dataset used for training, without doing any hyperparameter tuning.
This approach is quite different from what we’re used to, because as a development team, our first instinct is to develop. However, like what Andrew is saying, it’s more important to debug at the source, which is the data. So instead of tuning the model, we find ways to improve and produce a higher quality dataset for training.
Becoming a data-centric team is one of our goals for our project momentum. We want to empower everyone in our team to become more data literate. We want more of our team to contribute to refining our training datasets and test datasets, which we fondly call our TRDs and TEDs.
3. Annotation is critical to success.
Even the most revered datasets have errors. Often, it’s not the quantity of data that makes a real impact on the AI, but the quality with which it was prepared. That is why labeling is important. One wrong label can affect the result of the entire dataset.
The most successful AI teams are equipped with the skills that are required to execute machine learning from end to end, and yes, that includes annotation. Annotation is key, because this is where you are directly “talking to the AI”, essentially telling it what to learn. If you are careful in what you teach it – bounding boxes drawn correctly, objects properly identified, labels accurately placed – then you can be confident of its accuracy and performance in the wild.
Our team is gradually doing a shift on how we train. We are becoming more hyperfocused on our TRDs, ensuring that enough time is spent understanding what is required. This informs how we annotate, and in turn, produces more meaningful iterations and pushes project momentum forward.
ML model training is a very iterative process, which means that success is based on how fast you can go through the cycle of improvement.
I am very grateful to have had the opportunity to discuss, debate, and learn from the best minds in the industry, and to hopefully pass on the insights I have gained to other geeks like me.